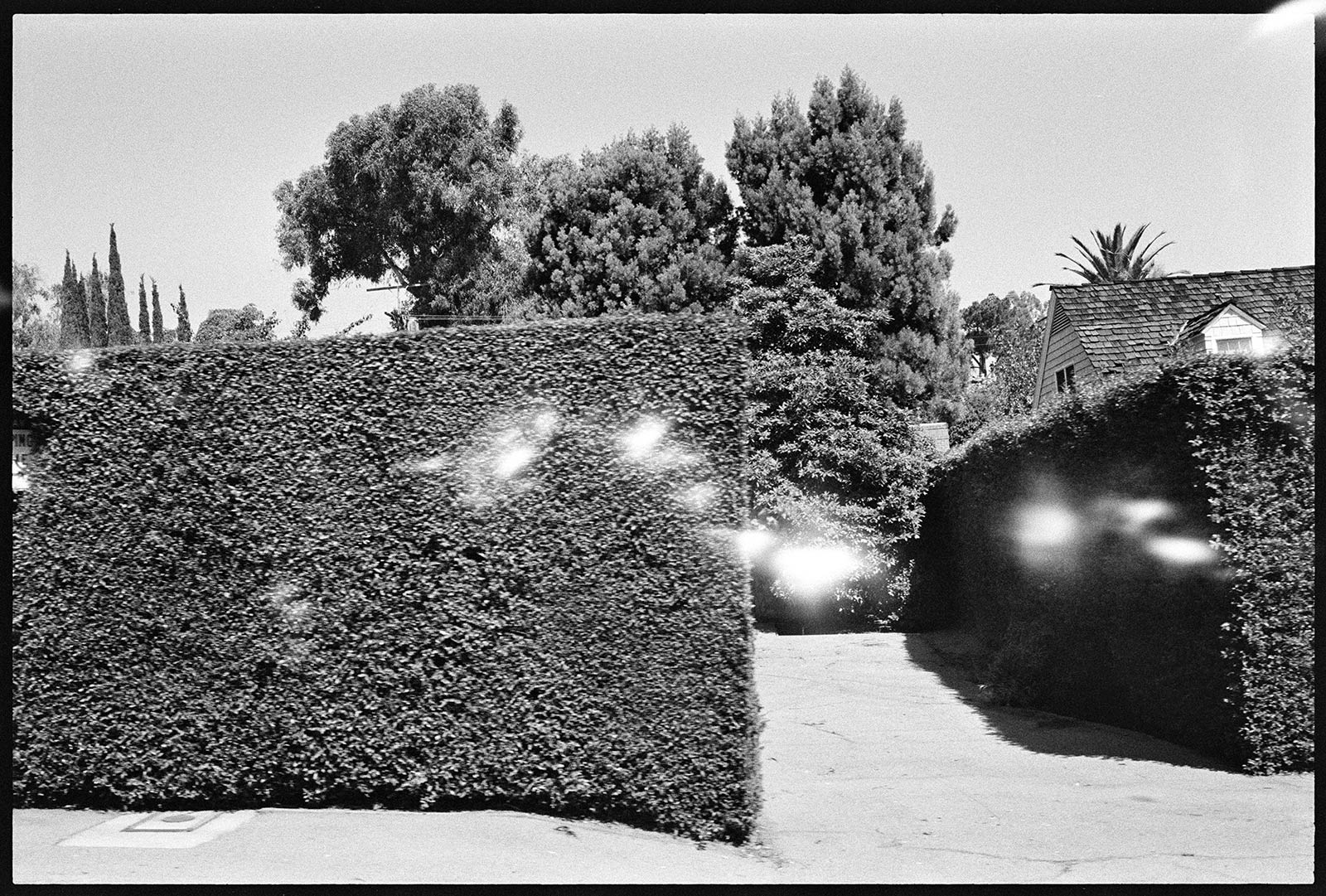
The high contrast between pavement and foliage, along with irregularities in the negative, may be why the computer identified “snow” in this image. Shoot from Sunset Boulevard, 1990, Ed Ruscha. Negative film reel: Gretna Green headed west (Image 0021). Part of the Streets of Los Angeles Archive, The Getty Research Institute. © Ed Ruscha
The first time I realized the computer, or more accurately the algorithm, was up to something funny was when it told me that among the 65,000 images of Sunset Blvd., there were over a thousand pictures of snow.
This seemed like an obvious error, but it underlined the joys and complications of working with an artificial intelligence (AI) to analyze over 60,000 images of Sunset Boulevard taken by Ed Ruscha from 1965 to 2007 as part of an initiative to digitize and make them available.
Indeed, I couldn’t be certain there was no snow in the photos. I hadn’t seen all the images. Even the photographers who digitized the negatives can scarcely claim to have “seen” the images. Working over the course of a year, two photographers used a custom-built imaging rig to capture over 120,000 photographs, typically at a rate of 100 per minute (with breaks in between for processing and coffee). Then we used the Cloud Vision API, a Google product to add additional metadata to the images—information that helped describe each image to make it findable when searching. No human eye saw them then either.
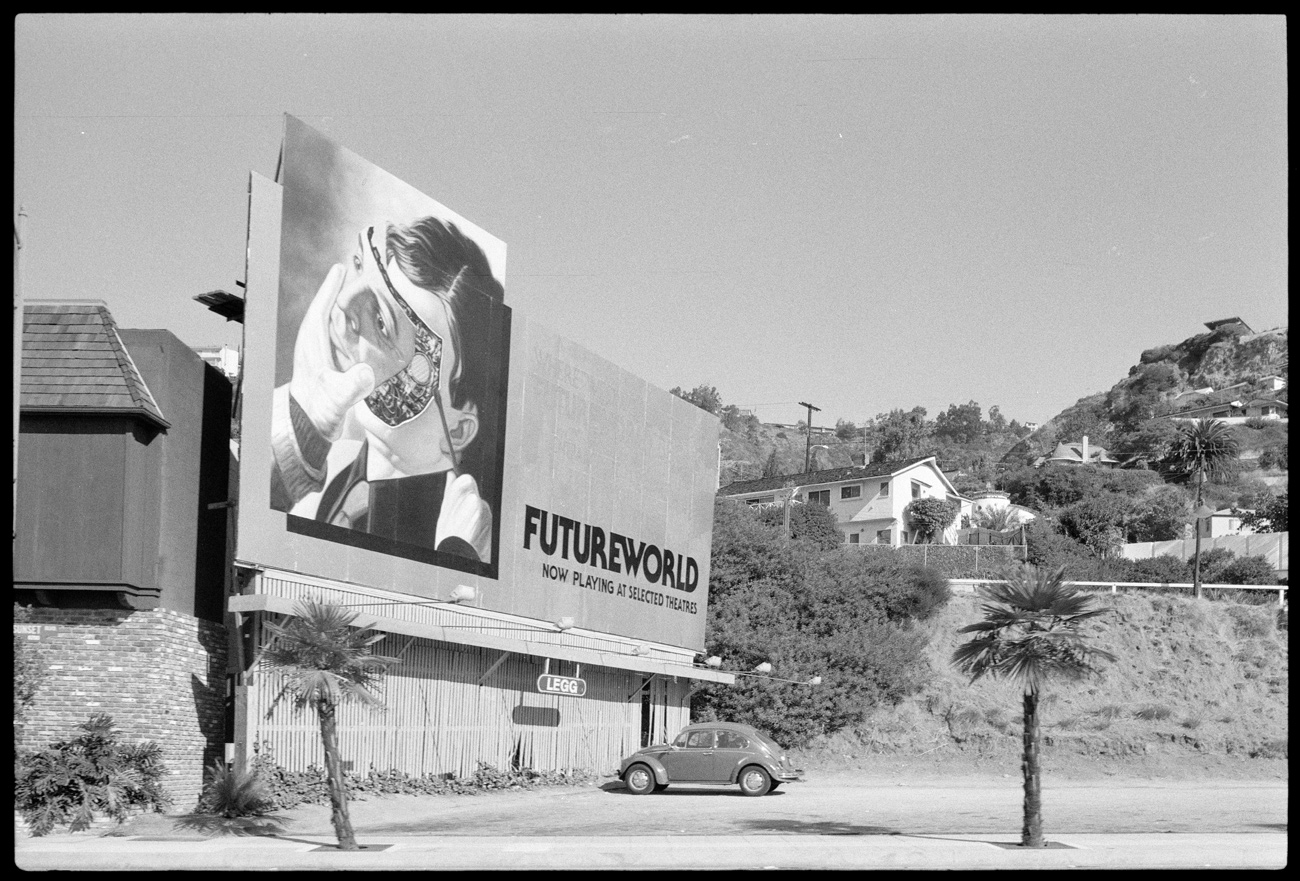
It’s unclear why this image was given the “snow” tag, but perhaps it was due to the white sidewalk. Shoot from Sunset Blvd, 1976, Ed Ruscha. Negative film reel: 8117 headed west (Image 0077). Part of the Streets of Los Angeles Archive, The Getty Research Institute. © Ed Ruscha
Computer vision is a process by which machines “look” at the pixels in an image and try to decide what objects or themes appear in them. The way it works is, as one might expect, complicated. But the basic idea is that if you show the computer an image of a cat, then tell the computer it’s a cat, the computer can, after a few hundred thousand examples, make a pretty good guess if a picture it’s never seen before has a cat in it. (Here’s a handy New York Times article on the topic.) Then repeat for dog, car, house, and so on, and the computer learns to find each new thing. When we processed the Ruscha images, the computer “saw” over a thousand unique things from “alley” and “almshouse” to “windshield” and “wire fencing.” No cats, though the Optical Character Recognition did detect the word “cat” thirty-seven times.
Does the computer do a good job of seeing? In our experience, the answer is, sort of! When you consider what the computer is doing and how fast it is doing it, the results are astounding. If you compare it to a human, the results can be mixed, but are almost always compelling in some way.
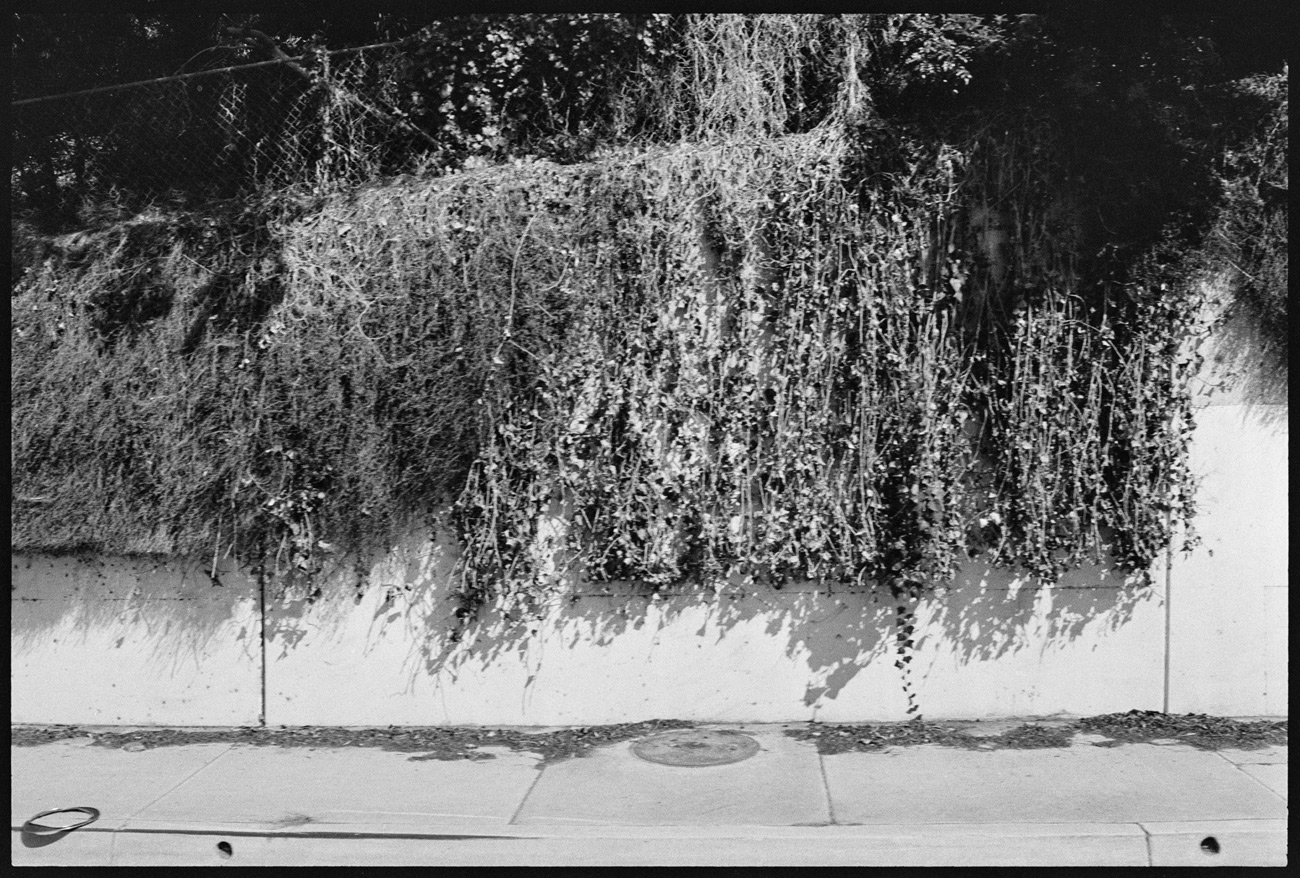
The computer could have tagged this image with “snow” because of the white retaining wall. Shoot from Sunset Blvd, 1990, Ed Ruscha. Negative film reel: Delfern headed west (Image 0016). Part of the Streets of Los Angeles Archive, The Getty Research Institute. © Ed Ruscha
It’s hard to say why the computer saw so much snow in the pictures. Sometimes referred to as “the black-box problem,” it can be difficult to explain the choices made by advanced algorithms, even more so when using a pre-trained commercial system as we did. Understandably, this is a growing concern for the research libraries and cultural heritage institutions like Getty who are using these systems to generate metadata to be used by their patrons.
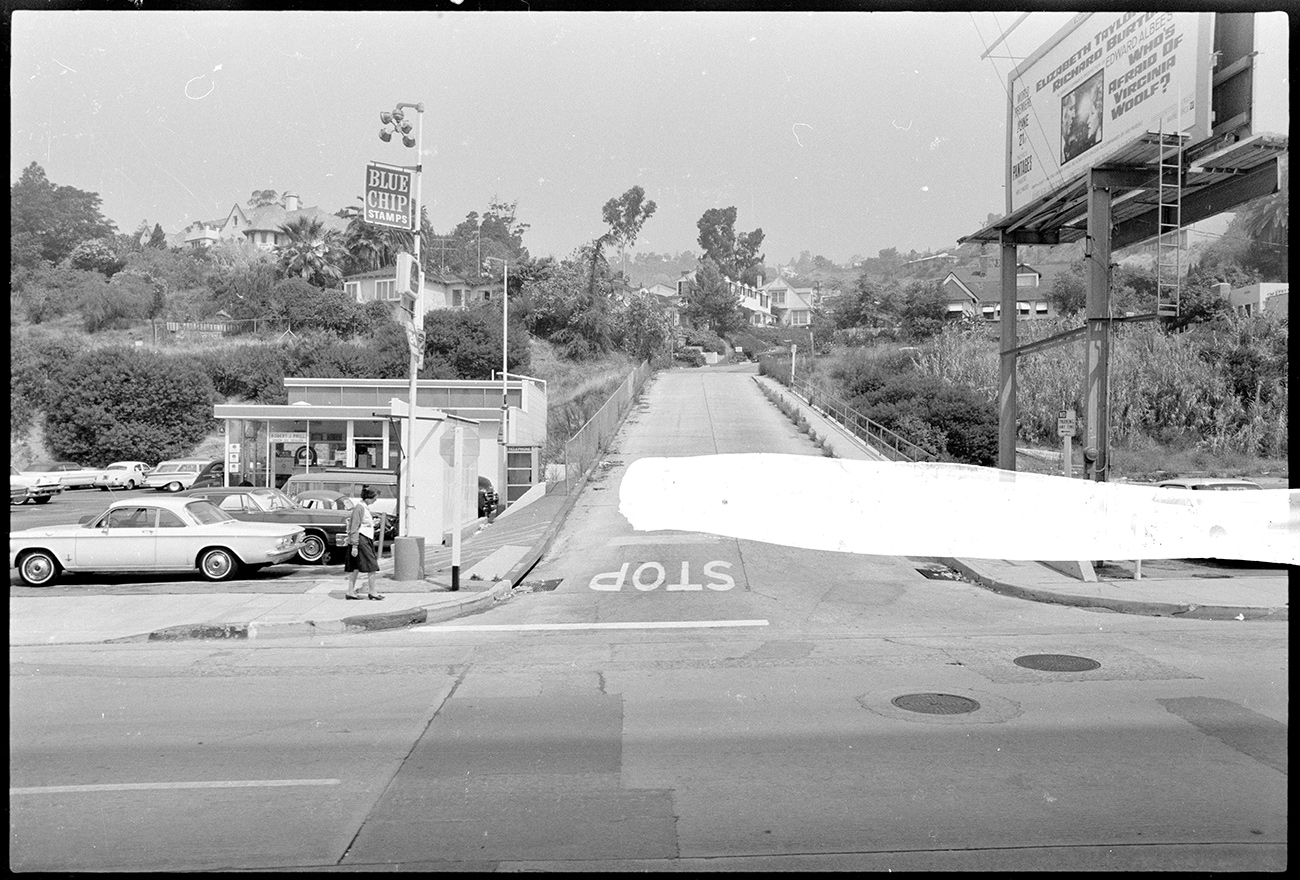
Ruscha marked this negative. The computer may have identified the white mark as “snow.” Shoot from Sunset Blvd, 1966, Ed Ruscha. Negative film reel: 8609 headed east (Image 0017). Part of the Streets of Los Angeles Archive, The Getty Research Institute. © Ed Ruscha
One of the beautiful aspects of the Ruscha collection is that, thanks to the sheer volume of images and the more than 100,000 tags it produced, we have a unique opportunity to see multiple examples of a tag (like “snow”). On its own, we might not be able to guess why the computer told us an image of Los Angeles contained snow. But when we look at the thousand images of Los Angeles streets the computer labeled with “snow,” we can start to piece together our own theories about the opaque inner workings of the technology. For example, a major commercial application of computer vision is self-driving vehicles, so if the system tends to be a little cavalier when it comes to identifying snow, the risk of a false negative (failing to see snow when it’s there) could be catastrophic.
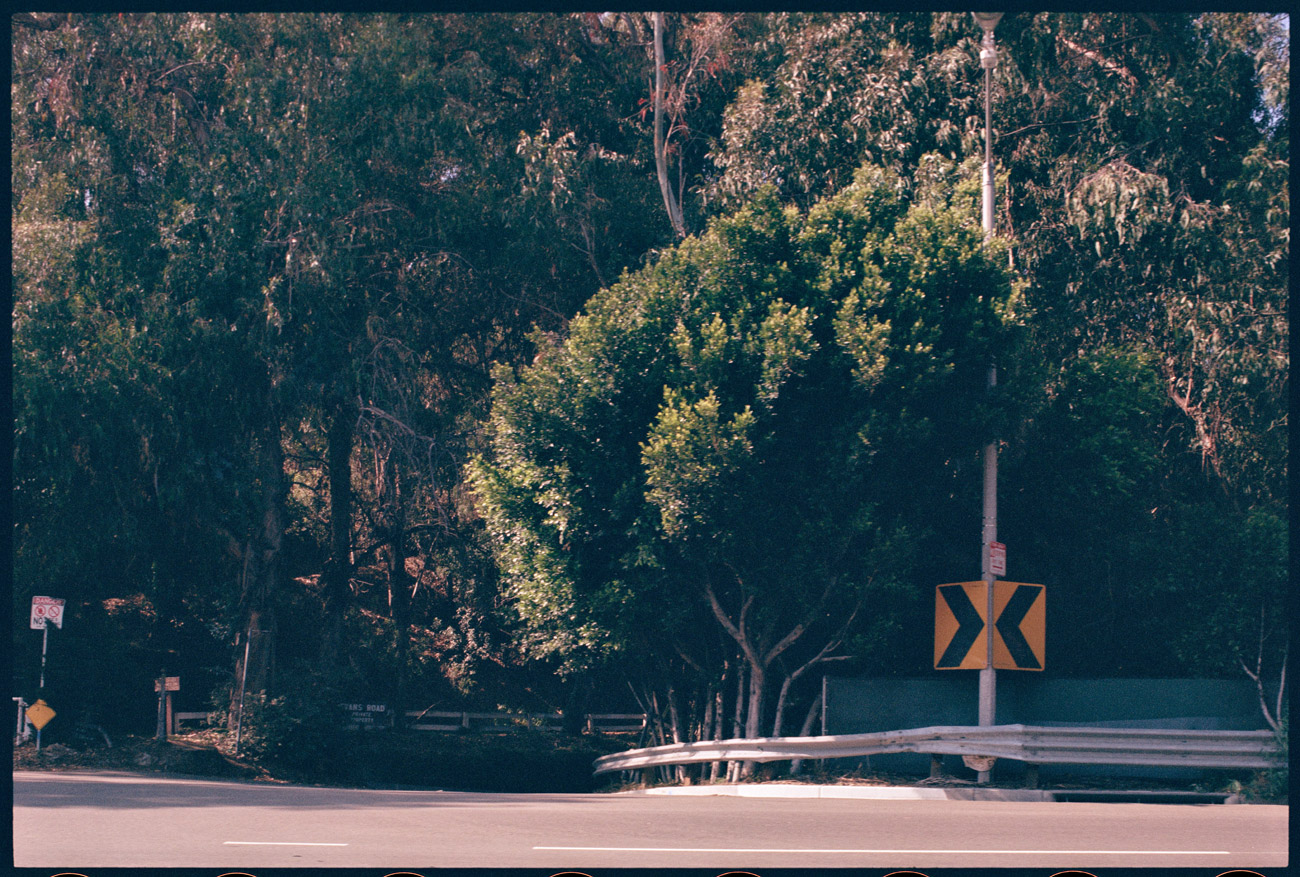
The computer may have picked up on the white curb as “snow.” Shoot from Sunset Blvd, 2007, Ed Ruscha. Negative film reel: Brooktree headed east (Image 0075). Part of the Streets of Los Angeles Archive, The Getty Research Institute. © Ed Ruscha
A slightly more scientific analysis that involved manually verifying the accuracy of the tags on a small subset of images suggested that the overall accuracy is around 80%. Again, astounding in the broad view but also probably insufficient for research, given that the most frequently valued quality of metadata is its accuracy.
As those familiar with Ruscha’s other works know, words often feature prominently in his work. To me, the brilliance of Ruscha’s art is that the choice of words, the lettering, and his paintings’ backgrounds all conspire to challenge my assumptions about how I visually process the world around me, revealing the hidden machinery that makes meaning out of what I see.
The mystery of what and how a computer sees seems right at home with Ruscha’s project, and is worth remembering as we begin to make computer vision work for our institution and our users. Just like with Ruscha’s work, every new output we encounter provokes reflection, growth, and a deeper understanding of a complex world.
Comments on this post are now closed.